Urban Forest Inventory Using Artificial Intelligence
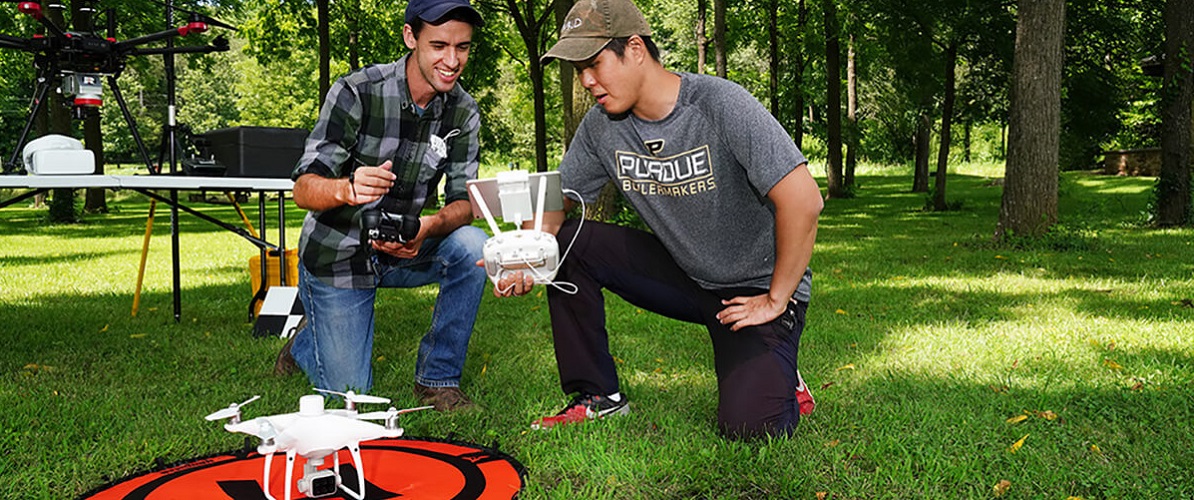
URBAN FOREST INVENTORY USING ARTIFICIAL INTELLIGENCE
Urban and community forests provide critical ecosystem services (e.g., air and water regulation) for human health and well-being. However, they are challenged by the changing climate, including drought and heat.
To improve the management of urban forests to better adapt to climate change, a first step is to understand the composition, abundance, and distribution of trees better in a given city. However, manually inventorying and quantifying the benefits provided by urban trees is prohibitively labor-intensive and expensive for most cities, limiting their ability to do strategic planning and manage urban forests.
This project’s primary goal is to automatically identify urban trees and quantify their ecosystem services by developing Artificial Intelligence (AI) algorithms that analyze remotely sensed data for major cities in Indiana.
Principal Investigators
Jinha Jung | Assistant Professor of Civil Engineering
Songlin Fei | Professor and Dean’s Chair of Remote Sensing
OBJECTIVES
Research using feature-based high-resolution classification on multi-temporal data for planned and unplanned disturbance, including fire, wind-throw, and logging.
Generate training data for urban tree identification.
Develop a deep learning algorithm to automatically identify individual urban trees’ locations and quantify their biomass using Indiana Statewide geospatial data products such as high-resolution LiDAR and aerial photos.
Quantify urban trees’ ecosystem services by coupling the individual tree biometrics with i-Tree, a state-of-the-art ecosystem service calculator.
RESOURCES
GDSL Online Data Portal for Indiana – 2017-2019 LiDAR DAtabase